ABSTRACT
The evolution of text generation has been revolutionized by the rise of transformer-based models. This has brought about significant changes in various fields, including news, social media, and scientific research. However, there is a need for a co…
See full abstracts, authors, references, citations & other publication information.
+
…mprehensive review that covers the historical evolution, challenges, and potential solutions in this domain. To address this gap, we have conducted a thorough survey that provides a comprehensive overview of text generation. We also investigate text degeneration, providing insights and mitigation strategies. Our survey sheds light on the current landscape of neural text generation, identifies forthcoming challenges, and highlights research areas that require exploration within the academic community.
Full Text/Reference Website: https://www.cscjournals.org/library/manuscriptinfo.php?mc=IJCL-136
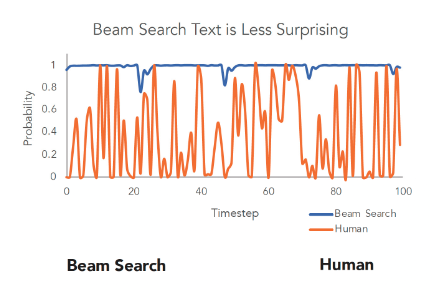
AUTHORS
Professor Christopher Crick – Department of Computer Science, Oklahoma State University, Stillwater, OK 74078 – United States of America
Miss Elham Madjidi – Department of Computer Science, Oklahoma State University, Stillwater, OK 74078 – United States of America
KEYWORDS
Natural Language Processing, Text Generation, Neural Text Degeneration, Large Language Models, Decoding Technique.
Indexing Keywords: A Survey on Neural Text Generation and Degeneration, Neural Text Generation and Degeneration, Neural Text Generation, Neural Text Degeneration, Text Generation and Degeneration.
Pages: 19-35
Revised: 30-08-2023
Published: 01-10-2023Published in International Journal of Computational Linguistics (IJCL).
Volume: 14
Issue: 2
Publication Date: 01-10-2023
*Randomly selected references used in the publication “A Survey on Neural Text Generation and Degeneration”.
- Ackley, D. H., Hinton, G. E., & Sejnowski, T. J. (1985). A learning algorithm for boltzmann machines. Cognitive science, 9(1), 147-169.
- Brown, P. F., Della Pietra, S. A., Della Pietra, V. J., Lai, J. C., & Mercer, R. L. (1992). An estimate of an upper bound for the entropy of english. Computational Linguistics, 18(1), 31-40.
- Brown, T., Mann, B., Ryder, N., Subbiah, M., Kaplan, J. D., Dhariwal, P., Neelakantan, A., Shyam, P., Sastry, G., Askell, A., et al. (2020). Language models are few-shot learners. Advances in neural information processing systems, 33, 1877-1901.
- Celikyilmaz, A., Clark, E., & Gao, J. (2020). Evaluation of text generation: A survey. arXiv preprint arXiv:2006.14799.
- Chen, S. F., & Goodman, J. (1999). An empirical study of smoothing techniques for language modeling. Computer Speech & Language, 13(4), 359-394.
- Cho, K., Merrienboer, B., Gulcehre, C., Bougares, F., Schwenk, H., & Bengio, Y. (2014). Learning phrase representations using rnn encoder-decoder for statistical machine translation. In EMNLP.
- Choi, Y. (2018). The missing representation in neural language models. In 3rd Workshop on Representation Learning for NLP (RepL4NLP).
- Chowdhery, A., Narang, S., Devlin, J., Bosma, M., Mishra, G., Roberts, A., Barham, P., Chung, H. W., Sutton, C., Gehrmann, S., et al. (2022). Palm: Scaling language modeling with pathways. arXiv preprint arXiv:2204.02311.
- Chung, J., Gulcehre, C., Cho, K., & Bengio, Y. (2014). Empirical evaluation of gated recurrent neural networks on sequence modeling. In NIPS 2014 Workshop on Deep Learning, December 2014.
- Cohen, P. R. (1979). On knowing what to say: Planning speech acts. Ph.D. thesis, ProQuest Information & Learning.
CITATIONS
Citations used in the publication “A Survey on Neural Text Generation and Degeneration”.
Currently there are no citations collected for this publication at scholarlyabstracts.com.
-
CONTACT US
Please feel free to us at scholarlyabstracts@gmail.com if you wish to:
- Get your journal, conference or thesis, registered with us.
- Update this publication content.